AI’s Hidden Environmental Footprint
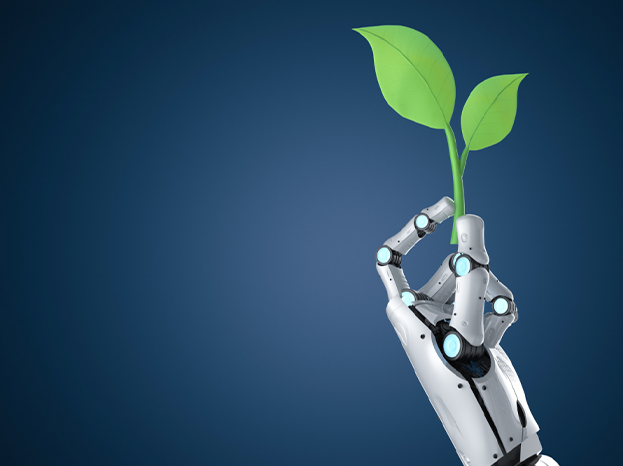
Climate change always brings images of thick, dark smoke billowing from factory chimneys, obscuring the once-blue sky. It’s natural for our minds to associate climate change with something ‘tangible’ that our senses can perceive, such as exhaust fumes of packed traffic in Delhi or smog over Shanghai. However, amidst the apparent culprits, there are some hidden or ‘intangible’ agents causing environmental damage without much recognition or awareness. Today we are experiencing a revolution with AI, Machine learning, Blockchain, and other ground-breaking technologies taking the world by storm. At first glance, these technologies might seem eco-friendly due to their lack of obvious mechanical or physical processes leaving any carbon footprint. But in reality, AI and other technologies have a profound impact on the planet’s ecology and climate. Take, for instance, the mining of Bitcoin — the most popular cryptocurrency — which requires an incredibly fast computing processor called GPU (Graphic Processing Unit). The use of these GPUs consumes a substantial amount of energy and as more people mine Bitcoin, the algorithm becomes more complex and hence requires even more computational resources. To put things into perspective, the energy consumed annually to mine Bitcoin surpasses the entire energy consumption of a country like Argentina with a population of 45 million¹. Another booming industry, Generative AI or Large Language Models (LLMs), shares similar computing needs. Training these models requires multiple instances of GPUs to handle billions of calculations within seconds, resulting in massive energy consumption. Additionally, Data Centers where these models are trained, require a huge amount of water to cool the equipment — similar to your cars needing a coolant to keep the engine cool. AI’s ecological toll Research from the University of Massachusetts Amherst² indicates that training only one Transformer model (with a neural architecture search) generates approx. 350 tonnes of CO₂. That is the equivalent of 205 return flights between New York and London! Another similar 2022 study³ on the carbon footprint of training a 176 billion parameter LLM called BLOOM estimated that 25 tonnes of CO₂ was generated in training that model. The following chart, reproduced from Stanford University’s Human-Centred Artificial Intelligence (HAI) Institute’s 2023 AI Index report ⁴, illustrates the CO₂ emissions from various studies. Notably, the GPT-3 model stands out as the biggest carbon emitter at 500 tonnes. The average power consumption to train GPT-3 model was estimated to be 1,287MWh — enough to power an average American home for 120 years. Image Courtesy of Stanford University’s HAI Institute. 2023 AI Index report Beyond carbon emissions, another pressing issue lies in the water footprint of these AI models. A 2023 study reveals that training the GPT-3 model in Microsoft’s U.S. data centres consumes a substantial 700,000 litres of clean, fresh water. This consumption increases to 3.5 million litres when the offsite water footprint (e.g. to generate electricity used for training) is taken into consideration⁵. This high water consumption is a cause for concern, especially as interactions with LLMs increase, leading to greater demands on water for data centre cooling. In other words, ChatGPT needs to ‘drink’ a 500ml water bottle for an average session of 20-50 Q&A⁵. Future of Research While AI holds great promise in optimizing energy usage and aiding in carbon emission reduction, it also poses a significant threat to the environment and its limited resources. Raising awareness about the potential environmental impact of these technologies is crucial, and organizations training LLMs should provide more transparency regarding their energy usage and emissions. AI’s presence is undeniable, and it will undoubtedly reshape how we work, interact, and innovate. Nevertheless, world leaders, tech experts, organisations and regulators must act urgently by supporting research institutes to conduct independent studies on AI’s environmental impact. Currently, the cost of training models for such experiments prohibits the effective study of the impacts. Establishing a comprehensive framework to achieve a Net-Zero AI footprint is also essential, considering the rapid progress in Generative AI development and usage. Remember, when it comes to the environment…we don’t have a Plan B
An SME CFO Guide to Digital Transformation
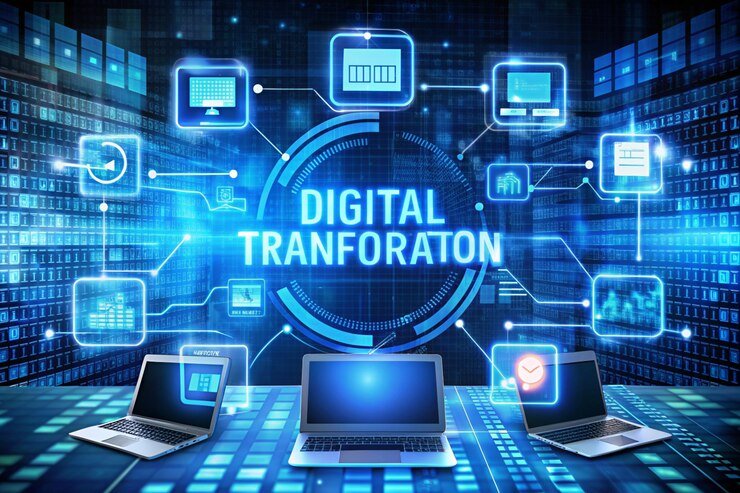
Digital Transformation is often considered synonymous with Digital native organizations, i.e. Googles and Amazons of the world, or at most something to do with the ‘top end of town’ or ‘blue chip’, global multinationals. Small and Medium Enterprises (SMEs) tend to be sceptical about this as it is either seen as a cost and resource-intensive exercise or regarded as something that will not deliver enough value due to the relatively smaller size of the SME. When it comes to the Finance functions of such SMEs, it becomes an even bigger challenge owing to the limited budgets and lean teams of a supposedly ‘back office’function. SMEs are the backbone of any economy. As per a report by the World Economic Forum, SMEs contribute up to 70% to global GDP and employment. The good news is that, in another report, 85% of SMEs realize the need to transform digitally to increase the efficiency of their operations, reduce costs and create competitive advantage. It is also one of the most frequently asked questions I get from CFOs and Finance Leaders during my keynotes on Digital Transformation — how can a small-sized organization afford to embark on a Digital Transformation journey? In this article, I will share FIVE steps that CFOs of SMEs can take to transform their functions Digitally. While at it, I will also bust some myths about why investing massive capital and resources has little correlation with the success of Digital Transformation. Assess current maturity: The first step is to engage with wider business to understand the current digital maturity of your SME. For an SME, a peculiar advantage is their rather closely-knit organization. Functional silos are less defined as opposed to their counterparts in bigger corporations. This can work to their benefit by approaching digital transformation needs from a business perspective as opposed to a standalone finance function point of view. To achieve that, the first step is to understand the current maturity of the organisation and identify the biggest areas where Digital will deliver maximum business value. This needs to be a holistic exercise across the organization and must not be restricted to Finance only. SMEs have the further advantage of being agile and less bureaucratic, so mapping the biggest value drivers of automation can enable an end-to-end business case of digitalisation. For example, to create a seamless sales funnel for your B2B customer, you might want to invest in not just the front end of the sales portal, but how it integrates with the back end from invoice processing, discounts, claims and payment management. This assessment will help you focus and prioritize your efforts on only the biggest challenges with the highest ROI potential of digitalisation. Clear roadmap: Once the maturity assessment is done and the key business challenges and areas of improvement are identified, create a clear roadmap on what, how and when to solve. Do not tackle everything at once. Start with small 3-6 month sprints of projects that have the potential to deliver maximum value. Look at the initiatives end-to-end rather than just stopping at the edge of Finance functional boundaries. If reporting ROI on Ad-spend is a big challenge, do not just focus on finding a technical solution for transaction processing. In fact, involve the Head of Marketing from the beginning to find an end-to-end solution for transaction processing, data ingestion and visualization. Firstly, this approach creates broader ownership of digital initiatives, and secondly, the organization-wide synergies of digital solutions help lower the costs of these initiatives. Leverage Cloud and Low/No-code tools: The exponential increase in cloud computing power and the corresponding decrease in cost have made the development, deployment, and widespread adoption of digital tools much more accessible than ever. With this relative ease of access to cloud computing and storage at a fraction of the cost of an on-premise server — coupled with their ‘speedboat’ advantage — SMEs can move much faster with digital transformation compared to many established firms. Use low and no-code tools like Microsoft Power Apps, Microsoft Power Automate Google’s App Sheet etc. For instance, Google App Sheet has built-in templates to create fully functioning CRMs, journal workflow automation, and Inventory Management apps. Similarly, for Data Preparation and extraction, SMEs can use Power Query, Power BI Datasets etc. depending on their size and needs. These are just a few examples of how today SMEs can leverage low-cost Cloud and Low/No-code tools to build an entire tech stack without having a single on-premise server or deployment. Start with Quick-wins and MVPs Although it holds for all organizations, irrespective of their size, starting small becomes even more relevant to SMEs. Utilization of the restricted budget to its maximum is the key to ensuring CFOs can score more budgets and solicit executive buy-in. Start with micro-projects that create a big impact. A simple dashboard on Power BI visualizing daily sales, receipts from customers, payments to suppliers, cash-in-hand, and Inventory levels can create a huge impact. To start, you do not need to establish a full-fledged Data foundation. Data sets in Power BI would work just fine. Similarly, using Microsoft Power Platform tools, you can simplify and streamline the month-end closing management process fairly quickly. Quick wins unlock an important aspect where the teams start to see the real tangible benefits of automation right away and hence get mobilized behind the digital transformation. For a digital transformation success, having an engaged team, that believes in improving processes through automation, is of vital importance. Choose success Partners wisely No matter how much we try to embed Transformation into ‘business-as-usual’ the daily grind does take over. Sometimes it either seems a bit too much to do with an already lean team or it might not be the core strength of your finance function. In that scenario, it will be wise to choose a success partner who can help advise you on your Digital Transformation journey. Do not make the mistake of taking everything on your own. Partnering with outside experts can help you get an
Beyond AI: Investigating The Algorithmic and Data Bias
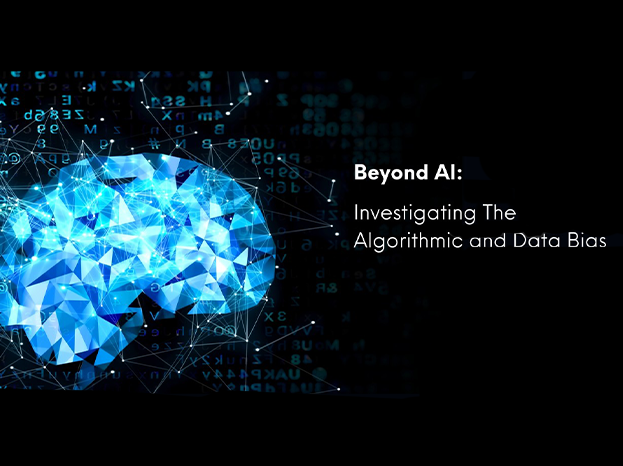
Recently Buzzfeed published an article featuring AI-generated images of Barbie dolls from different Countries. The images were generated using the famous generative AI model — MidJourney. The images created a huge backlash on X (formerly Twitter) as the majority of these images depicted massive biases and stereotypes. For instance, a South Sudanese Barbie was holding a gun and a German Barbie was reminiscent of a Nazi soldier. The article seems to be removed from Buzzfeed since then. Algorithm bias is very real and poses an even bigger threat than human bias. We tend to ‘legitimize’ or ‘validate’ the results and our thinking with technology. The ‘unconscious’ bias that algorithm carry can remain undetected and work in the background to create a systemic unfair advantage or disadvantage for a specific group of people. The worst part is how quickly it can multiply and scale as more and more data with biased results is consumed by the algorithm. In a recent study by Researchers at AI Start-up Hugging Face and Leipzig University generated 96,000 images using Stable Diffusion models. The results have been pretty disturbing, if not unsurprising. For instance, when tasked with crafting images portraying CEOs or Directors, the models predominantly generated images of white males. They have since then made this study available to be used by the public in the form of an interactive tool here. In the past, Amazon had to shut down its AI-based recruiting tool as it was showing bias against women candidates. Similarly, in 2016 Microsoft launched a Twitter Chatbot named Tay for casual and playful interaction with users. However, within 24 hours, it ‘learned’ from public comments and interactions and started tweeting racist and antisemitic comments. It had to be shut down! With a vast population being exposed to AI today, in one form or the other, it is critical as part of our Digitial literacy to understand the Algorithm and Data Bias. This ensures we are not taking the results generated by the likes of ChatGPT at its face value and doing sufficient human-conscious and contextualised fact-checking. Algorithm Bias Kate Crawford, a Distinguished Research Professor at New York University explains AIgorithm bias in terms of Allocative and Representative Bias. Allocation Bias occurs when the algorithm unfairly allocates an opportunity to a specific group e.g. Amazon’s recruitment algorithms favouring Men over Women. On the other hand, Representative Bias happens when the algorithm stereotypes or ‘represents’ a certain group with a specific trait. For instance, COMPAS, a widely used algorithm in U.S. criminal justice system is found to be representationally biased towards black defendants. It is far more likely to flag a black defendant as having a higher risk of recidivism than a white defendant. Data Bias Another aspect of bias in AI models is caused by Data. For simplicity’s sake, I will broadly categorize this into two types; Source and Diversity. LLMs use a vast amount of previously human-generated data as input for training. The ‘source’ and content of the data these models are consuming are mostly unknown and in most cases proving to be detrimental to the neutrality of AI algorithms. Take the example of MidJourney. The company is under heavy criticism for massively infringing copyrights as it is training its models on a huge data set of previously human-generated images. The source and biases associated with these images are unknown and how it will systematically include or exclude certain groups of people unfairly is also a subject of debate at this stage. Similarly, if the algorithm is being trained on a set of data that is not representative of the population, it creates tendencies and results which again are skewed towards a specific point of view. The earlier example of Tay by Microsoft is a case in point. The data is not Diverse enough to provide us with representative results. Aftermath Stanford HAI recently reported based on The AI, Algorithmic, and Automation Incidents and Controversies (AIAAIC) database that over the past 10 years (2021-2012), the number of ethical issues associated with AI has increased by 26 times i.e. from 10 cases to 260 cases. This increase in ethical concerns is a direct result of a lack of responsibility in AI algorithm development and consciously or unconsciously introducing biases in the AI models. A recent study by Bloomberg used Stable Diffusion, a text-image AI model, to generate 5,100 images of people as a representation of workers for 14 jobs. The analysis suggested that the images generated for people working in high-paying jobs (Lawyers, Engineers etc.) were mostly of lighter skin tones, while images of people with darker skin tones were predominantly generated for prompts like “Janitors” and “Dishwashers” etc. This is far from reality. The same report compares how many ‘Women’ images were generated for the keyword “judge”. It was only 3%, while in reality, 34% of US judges are women! How this will change the worldview we hold today…you guessed it right…is yet to be known! What is next? A report by Gartner indicates that by 2025, 30% of marketing from large organizations will be through AI-generated content. Another report by Bloomberg suggests that the Generative AI market is set to reach $ 1.3 trillion by 2032. This puts a huge responsibility on large organizations, Governments and Academia to join hands to fight for ethical and responsible AI practices. A global standard providing Data Lifecycle frameworks to validate data sources and their authentication needs to be introduced. Blockchain can play a major role in this data governance and modelling by providing an immutable, distributed and trusted network to validate content generated or consumed by AI models. As for the general public, as I always advocate, improving Digital Literacy is the only way to make informed choices with the use of AI. The core definition of Digital Literacy is no longer how you can use a computer, but extends to how we interpret, understand and connect the results generated by AI algorithms. With the power to reshape perspectives, AI holds the key to forging a brighter future – provided we remain vigilant and aware of its limitations.
Data isn’t ‘the new Oil’ — Digital Talent is!

A metaphor famously associated with famous British Mathematician, Clive Humbly…”Data is the new Oil” has been around for some time. However, we generally miss the subsequent part which implies that similar to how Oil is only valuable in its refined form, Data needs to be distilled and broken down to have any value. In crude form it is just that…Data. Even more unsettling is the common notion that through an advanced analytics tool or AI/ML deployment, we can extract the true value from the Data. Imagine having cutting-edge equipment and technology to refine Crude Oil but not having the skilled Engineers or Operators to run the machines. Surely, there is not much that can be extracted from the Crude Oil. Companies are facing a similar dilemma today. Over last 10 years, an estimated 500 zettabytes of Data has been generated (Source: Statista) . Organizations are sitting on troves of such a highly valuable resource and are in a race to be the first to extract the true value from data. Internet of Things (IoT), POS consumer insights, and social media interactions are just a few contributors to this massive Big Data. On the other hand, in a rush to do so…majority of initiatives fail (some studies suggesting up to 87.5% failure rate!) to generate much value for the organizations. A key factor being lack of the Digital Talent. 2023 Future of Work report by LinkedIn states that “51% of US executives are excited about AI advancements, but do not yet know how their organization will leverage AI tools and skills.” Similarly in a SAS Survey report, 63% of respondent indicated the biggest skill shortage they are facing is in the domain of AI and Machine Learning. In fact, Netflix recently posted AI related positions paying up to $900,000 annually (Source: Sky News). It is mission-critical to have the right talent as we witness huge investments being poured into organizations’ AI/ML deployment efforts. A report by Grandview Research Inc. estimates the global AI market to reach $1.8 trillion by 2030! There is no easy solution to address this shortage of AI or Digital skillset. However, a sustained effort at both individual and organizational level can surely bridge this gap. Here are some of the ways Organizations and Individuals can enable this: Mid-career training programs Organizations need to create tailored mid-career training programs for their existing staff to upskill them with AI and Digital skills. This includes staff who is non-technical but are domain experts. Incentivize, sponsor, and facilitate top-talent through formal on-campus digital upskilling. Individuals on the other hand need to be committed to these learning and development programs. Degree vs Certifications There is a need to create a balance between roles requiring a college degree vs the ones for which certifications are good enough. Specialist roles like Engineering and Health professionals do require a college degree but digital skills like Data analytics, AI/ML deployment, and Data Science can be learned through doing various certifications. Platforms like LinkedIn Learning, edX, and Coursera has number of individual and professional certifications available in these fields. On-the-job training There is nothing better than old-school on-the-job training. Engage employees who are passionate about emerging technologies in exciting new Digital transformation projects. Encourage them to spend time on upskilling them through both exploring online resources as well as practical on-the-job problem solving. Digital Mindset and leading by example A study by BCG suggests that organizations with a digital culture and mindset are five times more likely to succeed in their digital initiatives. A top down culture of continuous learning and development needs to be adopted by organizations and individuals who are in leadership positions. Reverse or peer-to-peer digital mentoring is an excellent way to do so. Moreover, creating in-house learning academies, establishing reward systems for top learners, and doing train-the-trainer style programs are all great ways to enable organization-wide upskilling. When organizations invest in such initiatives they not only retain talents but also attract top talent. Digital is not only enabling creating an innovative consumer experience, but can also help organizations design the best ‘employee experience’. Organizations and Individuals who will invest in this will come out victorious in this war for digital talent.
Embracing the Tech Disruption: A Paradigm Shift in Corporate Finance’s Evolution
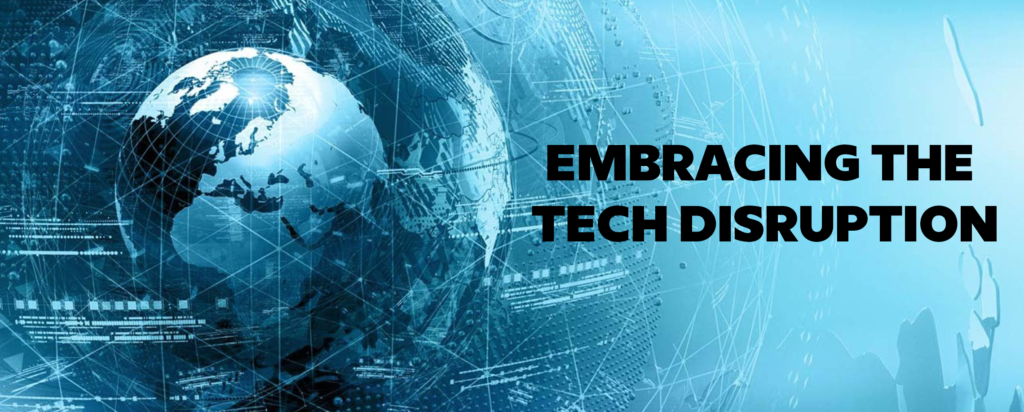
The first in a regular series of insights for CFO Magazine A/NZ on digital finance transformation from Tariq Munir, exploring key trends reshaping the Corporate Finance Function of the Future. There is no better analogy to describe the impact of technology on business than what Thomas Siebel refers to as a “Punctuation in the Corporate Evolutionary equilibrium”. An “Evolutionary Punctuation” is an idea that evolution occurs in spurts instead of slow and steady paths. This is what technology has done to the business world of today. A sudden spurt of innovation is reshaping the world in ways we could not even imagine a few years ago. Whether we call it a Punctuation or a Disruption, it is redefining and challenging the way we think, interact, and more importantly…create! The Transformation of Corporate Finance While technology is creating a compelling customer experience and reinventing business models, its most profound impact is on how business processes are being redesigned to adapt to these core changes in businesses. Corporate Finance lies at the heart of this technological upheaval. It is no longer merely a back-office support function; it is being transformed into a strategic growth navigator for organizations. The rapid digitalization of core finance processes, including transaction processing, consolidation, and financial reporting, serves as the biggest enabler in this transformation. In fact, Finance is one of the key areas experiencing a rapid increase in digital investment. A study by Gartner this year suggested that 92% of CFOs plan to increase their tech spend in 2023. It does not stop there…by 2028 it is predicted that 50% of businesses will be using AI to drive bottom-up planning. However, amidst all the noise and buzz surrounding AI and ML, Finance leaders are facing increasing challenges in prioritizing the right technologies to enable a digitized future. Allocating resources to the appropriate technology becomes crucial; otherwise, the dream of becoming true strategic business partners for Finance will remain just that: a dream! Five Innovative Trends in Corporate Finance In our study of trending and innovative technologies, we have identified five broader trends or innovations that, if invested correctly, can truly reshape and reinvent the finance function of the future. Data and Development Democratization Organizations that have truly unlocked the potential of technology, have transitioned from rigid on-premise Data warehouses to flexible cloud-based Data lakehouses. These Data lakehouses host more than just ERP systems and have data from both structured, unstructured, and semi-structured sources. Walmart, the world’s biggest retailer, created a state-of-the-art analytics hub called “Data Café”. This enables Walmart to model and analyze a huge volume of internal and external data at scale, including a massive 40 petabytes of transactional data. This democratization of data allows for the de-centralization of the reporting function traditionally associated with Finance. It empowers “non-technical” functions like sales and marketing to be self-sufficient in creating reports and dashboards. However, this self-serve revolution does not diminish the relevance of Finance; rather, it frees up time for Finance professionals to focus on strategic business partnering and data-driven decision-making. Furthermore, the democratization of data has given rise to a new breed of developers called Citizen Developers. These functional experts can now utilize low and no-code tools to develop fully functioning apps, enabling workflow automation, month-end process management, expense tracking, and more. For instance, Accenture trained their Citizen developers and generated 8,000 apps in less than 6 months, a task that would have otherwise taken 5 years! They also reduced dashboard reporting time by 98%. Tools like Copilot for Microsoft PowerApps are even taking it further by creating a ChatGPT-like experience to create fully functioning apps and workflows. Process Mining If there is one technology on which we can bet to revolutionize Corporate finance, it will be Process Mining. It is effectively a convergence of Data Science and Process Management. Process Mining delivers an agile business process optimization and more importantly, eliminates human bias from traditional interview-style process mapping exercises. An algorithm sits on the ERP and other systems and logs all the events and transactions. Based on these ‘event logs’ it creates process visualizations and then leverages, what J.C Licklider famously termed as, “Human-Machine symbiosis” to recommend the best possible optimization and simplification of processes. Suncorp Bank in Australia achieved massive efficiencies by partnering with Queensland University, reducing claim processing time from 30-60 days to an impressive 1-5 days. Further, incorporating process mining into a digital transformation strategy can deliver tangible benefits to a company’s bottom line. For instance, GE Healthcare, a global healthcare leader, streamlined its day-to-day operations and unlocked working capital efficiencies, resulting in an increase of $1.3 billion in free cash flow. Process Digital Twins Digital Twins have been employed by businesses for years, creating virtual replicas of physical products or systems. For instance, based on huge amounts of data generated by IoT sensors on a production plant, a digital model can be created for the whole plant which will then be used to simulate various scenarios to optimize processes like production planning and predictive maintenance. Countries like Singapore have created Digital twins at a Country level to help them with resource and urban planning. In Finance, using a similar approach like process or business mining we can create a Process Digital Twin of the entire Finance organization. However, the difference with process mining is around its scale and interaction with other business processes. A Financial process digital twin extends beyond a single process like Order to Cash or Record to Report. It captures the ‘digital-footprint’ and spans all the sub-processes, operating models and data flows within Finance processes and how they impact other business and financial metrics e.g. supply and demand planning, sustainability goals, productivity metrics and so on. In addition to creating visibility of product level information, this can revolutionize the way we make decisions around product lifecycle and how non-financial information is integrated, for example, with financial information to report on carbon footprint, profitability and other ESG measures. Generative AI This is by far the most talked about
Generative AI: The Future of Productivity

One thing is crystal clear: Artificial Intelligence (AI) is no longer a buzzword or science-fiction subject. A recent 2023 research by McKinsey suggests that AI can generate between $17.1 trillion to $25.6 trillion of annual value (The Economic Potential of Generative AI by McKinsey, June 2023), just shy of the US yearly GDP of $29 trillion and potentially higher than China’s GDP of $19 trillion (Source: IMF 2022 GDP report). Seizing the Generative AI opportunity Generative AI alone is expected to generate more than $7 trillion of annual economic value. This productivity will be unlocked across industries ranging from healthcare to manufacturing and retail. The economic value comprises of net savings from use-cases evaluated by Mckinsey and massive ‘knowledge worker’ productivity generated through the use of generative AI in automating, streamlining, and eliminating work In the latest 2023 Work Trend Index report by Microsoft, approximately 60% of work hours today are spent in emails, meetings, and chatting. This leaves little time to create and do meaningful work. Generative AI, with its ability to understand and respond to natural language, can partner with humans and possibly shift this balance towards more strategies, creative and innovative work. Governments and organizations can deploy generative AI functionalities and create real productivity gains through various use cases, including: Research & Development: From early ideation to the generation of test data and designs for physical and large-scale products, generative AI can significantly increase the speed-to-market and reduce the cost of repeated prototyping. Marketing and sales operations: From creating personalized conversion funnels to accelerating content writing for marketing campaigns, generative AI has the potential to significantly improve the Return on Ad spend. Consumer research: Analyzing massive amounts of data, creating and testing scenarios through the use of synthetic test data. Supply chain optimization: Converging with predictive analytics capabilities to recommend courses of action based on scenarios ranging from geopolitical to macroeconomic conditions. This enables taking pre-emptive measures to optimize supply chain and logistics planning. Contract and policy generation: Drafting legal contracts, negotiating deals, and co-creating new regulatory frameworks relevant to various industries. Risk management: Analyzing regulatory and control frameworks, matching them with transactions and documentation to identify possible breaches and rectification actions. Environment, Social, and Governance (ESG): Scrutinizing myriad regulations, relevant industry publications, greenwashing cases, and published ESG reports to help devise an optimal ESG strategy. These are only a few use cases, barely touching the massive potential generative AI presents. The AI space is generating excitement in both general users and investors alike. However, it is important to understand the far-reaching and broader impacts of this technology on the social, economic, and political landscape. Governments and organizations that already have a head start in deploying these technologies can create a huge economic and skill gap. To unlock the true potential, understanding these risks and creating a balance with the returns is of utmost importance. This might be our opportunity to create a more humanistic, balanced and accessible tech-enabled future for all.
Finance Transformation: The AI Dilemma
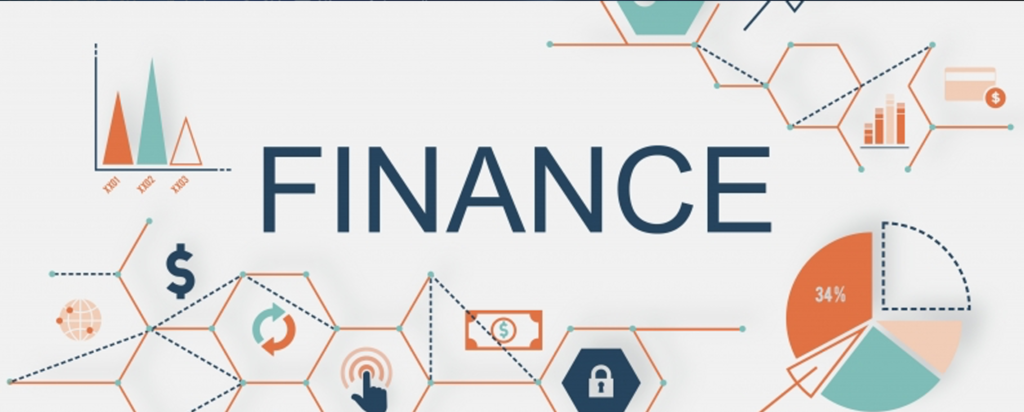
Former US Secretary of State Henry Kissinger famously said, “If you don’t know where you are going, every road will get you nowhere.” This is so true and apt when it comes to AI hype and how Finance functions are scrambling to find meaningful use cases. No conversation related to Digital Transformation is complete today, without references to Generative AI and Predictive analytics being part of it. AI provides a huge potential to transform the ways of working, redefine business processes and enable massive productivity. One can find numerous successful case studies where organisations have generated real efficiencies and cost savings using AI. For instance, a Global microchip manufacturer saved more than 5,000 hours annually just by deploying AI for their Accounts Payable Invoice processing. However, the dilemma lies in today’s predominantly ‘use-case driven’ approach. Finance FOMO? CFOs and Finance Leaders, in fear of missing out, rush to treat AI as a one-size-fits-all solution for Finance Transformation. This addresses some automation needs in the short-term but eventually results in a set of disparate AI deployments aggravating the Organizational ‘Technical Debt’. Gartner predicts that by 2024, half of Finance AI deployments will be either delayed or cancelled due to scale-up issues! The majority of business processes today including Finance came into existence as a direct business need during previous industrial revolutions which were dominated by mass manufacturing. In a way, the Finance processes today mimic a typical production floor’s assembly line. A systematic ‘handover’ of the output from one sub-process to the next…in other words a chain of interdependent sub-processes. One cannot start the Monthly Forecasting process before Month-end books are closed and books cannot close until all the cash receipts from customers are applied to invoices, for instance. Now these processes did serve well in the previous industrial revolution. However, since the 4th Industrial Revolution, Organizations have been quick in digitally evolving. An accessible and inexpensive Cloud Computing, coupled with AI, IoT and Big data, has re-invented the entire business model. In fact, new industries (e.g. ridesharing, SAAS etc.) came into existence that never existed a few decades ago. Unfortunately, the Finance and accounting processes have not been able to keep pace with this rapid technological and business evolution happening around it. Numerous recent studies suggest that up to 60-70% of Finance processes are still manual. So, the question arises; “How does Finance deploy AI and use the technology to transform its ways of working” ? The answer lies in a paradox! Instead of asking how AI will transform the Finance ways of working, we need to think about how we transform our existing ways of working to be able to deploy AI. This approach will not only promise scalability in the long term but will also ensure delivery of tangible productivity. CFOs and other Finance leaders should consider an ‘inside out’ approach to deploying AI. Here are the key considerations in adopting such an approach and ‘How’ you can start today with this approach: Start inside out Ironically, the most important step to automation does not start with technology. It starts with something more basic. Review the existing complexity and interactions within finance processes and the operating models supporting these processes. Simplify, streamline, and optimize these inter-dependencies. Automating complex processes only increases further complexity and hence results in most AI deployments becoming part of legacy systems. How you can start today: Create a Finance organization-wide “Simplification Campaign”. Create a professionally competitive environment between teams on who is driving the most simplification processes. Clearly measure the results in terms of hours saved or cost reduced. Reward the teams to encourage the right behaviours. Bring an outside-in perspective In addition to addressing the internal processes, Finance needs to bring a simplification or transformation perspective from its key business partner functions. For instance, we can simplify a process to record and pay media invoices, we also need to factor input from Marketing function on simplifying end-to-end process of vendor selection till receipt of services and payment. How you can start today: Involve key business partners on simplification journey right from the beginning. Sometimes, the biggest complexity lies at the edge of Finance rather than within the function. Deploying AI in Finance to automate the processing of invoices will end up in diminishing the returns over time as the linked processes outside finance are still complex and manual. Standardize & Scale AI from its evolutionary standpoint is still at, what Yuval Noah Harari recently referred to as an “amoeba of Artificial Intelligence”. Effectively what this means is, that AI is still primitive and only at the stage of doing specific tasks. The major difference between AI in the 1950s and today is the cheap computing power and access to huge amounts of data. In other words, we must understand that AI does not have a consciousness or ability to make decisions of its own (…not yet at least). Therefore, the success of an AI within the organization depends upon how standardized the processes are! The more your processes are streamlined and standard across different functions and business units, AI scalability becomes easier and easier. How you can start today: Create a core team of Process and transformation experts within the Finance teams who act as a Centre of Excellence. Share best practices across different business units or functions to ensure scalability. Consider setting up micro-digital factories consisting of a combination of ‘dedicated’ data analysts and ‘revolving’ functional experts. AI is a fascinating technology and the strides we are making today is changing the world as we know it. However, the rush to implement an AI-first strategy needs to be tackled in a careful way. Holistically evaluating technology requires a responsible and informed decision making process. Treat AI as a ‘Digital Employee’. Similar to how we would carry out an appropriate due diligence before hiring a physical worker, the digital worker requires the same…if not more! The true benefits of AI lies just beyond the edge of its hype and indeed…in its ability to be your co-pilot.
Is Generative AI the Future of Education?
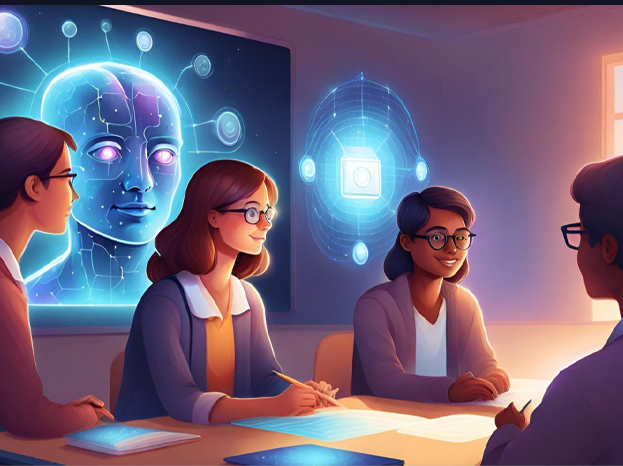
Imagine your 10th-grade Math class is happening in 2025. Apart from the usual dread of algebra, there is one peculiar feature. Right before Sam, the class teacher, starts the lesson, the iPads on students’ desks light up with a new notification “Tailored learning plan – 13 Apr 2025: Solving Differential equations”. What is unique about this is the ‘Tailored’ part. Instead of Sam coming in with a prepared lecture that she would deliver irrespective of the learning capabilities and needs of the students, she used Generative AI (GAI) to create a custom learning plan for each student. GAI analysed every student’s performance history and came up with a specific plan to ensure her teaching experience is not just a one-size-fits-all approach. Instead of teaching, it is now transformed into a ‘learning experience’ where Sam, her Students, and a GAI on their iPads are working in collaboration. Seemingly far away, something similar to this will become a standard mode of education in the near future. The rise of Large Language Model (LLM) based GAI is challenging the conventional teacher, student, and school relationship. A recent study by The Association of Heads of Independent Schools of Australia (AHISA) suggested that up to 80% of high school teachers have been using GAI to help them with lesson preparation. Similarly, a survey by Youth Insights showed that more than 70% of high-school students are using this tech. However, in stark contrast, a global survey of 450 schools by UNESCO revealed that only 10% of these schools have any GAI policy in place. While a school textbook is rigorously reviewed, published, and driven by national education policies…output by likes of ChatGPT and other GAI carries an inherent risk of misguidance. Students and teachers alike are prone to be subjected to data and algorithm bias. The tech dependency that it creates can be harmful to discourage students and teachers from carrying out independent research and encouraging creative or critical thinking. In addition to that, problems like lack of visibility in how a certain output is generated, algorithmic ‘black-box’, AI hallucination, and potentially unethical content are all real issues that need to be addressed before we can integrate GAI effectively into the educational ecosystem. One possible solution lies in developing Education-specific Generative AI LLMs or ‘EdGPTs’…as referred to in UNESCO’s latest Guide for Generative AI in Education and Research (Published July 2023). These LLMs are trained on specific high-quality and domain-specific data, rather than the entire internet, to exclusively serve education, research and pedagogy practices. In future, it might be just one model or a connected suite of more than one EdGPT specific to a domain of expertise e.g. EdGPT for Physics, or EdGPT for Clinical Psychology. Get ready to pay for an additional subscription as part of the current learning stack you have as a student, teacher or researcher! Although at a very early stage, the future is promising for EdGPT models. Here are a few developments happening in this space: East China Normal University has developed ‘EduChat’, an open-source foundation model to support teaching and learning. TAL Education Group is developing MathGPT which, as the name suggests, will focus on mathematics-related problem-solving and lecturing. South Australian Government is partnering with Microsoft to develop “EdChat”, which is a ChatGPT like LLM specifically for supporting teachers and students. This is a huge step, as such controlled experiments at the state level can help shape our understanding of the impacts GAI will have on learning experiences. KhanMigo is another remarkable example — an AI tutor by Khan Academy Unlike ChatGPT, it does not just give straight answers to the questions asked by a student e.g. on how to solve math problems. It rather provides them with guidance on how to solve that…more like a 1:1 tutoring experience. AI is here to stay and whether we like it or not, it will reshape the way future generations will interact, learn, and more importantly ‘create’. It is incumbent upon Governments, Educational Institutions, and Corporations to work collaboratively on a robust Global Policy Framework governing the development and use of GAI for education. UNESCO’s Director-General Audrey Azoulay Refers to Generative AI as offering tremendous opportunity but can equally cause harm and prejudice. She proposes a broad public engagement, and the necessary safeguards and regulations from governments before this can be integrated into the education system. Similarly, Professor and Chair in Digital Economy at QUT (Queensland University of Technology), Prof. Marek Kowalkiewicz recently said that while generative AI can be a problematic technology, that doesn’t mean we can ignore its existence. (Source: Linkedin news Australia) At the end of the day, education has the power to define the world. Leaving it in the hands of uncontrolled AI models can be devastating. However, channelling it in the right way can prove to be powerful and enable the democratization of education. Nelson Mandela once said, “Education is the most powerful weapon which you can use to change the world.” We must ensure this weapon becomes a catalyst of change for good to influence a positive and better future for all.
Results-driven Digital Transformation: The Power of going small
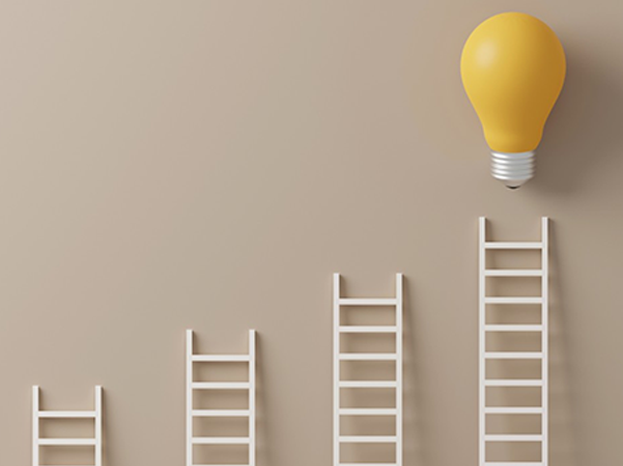
Committing significant resources and time is generally considered a preamble to success in any Digital Transformation journey. While having an overarching vision and a clear roadmap is critical, it is seldom beneficial to go all-in without a pointed digital investment and mobilizing the organization behind the digital efforts. Most firms get fixated on creating myriad projects involving cross-functional teams and resources. Unfortunately, after spending a number of years and a huge amount of budget, the majority of cases do not present much discernible benefit or progress. Gartner has predicted Global IT spending to reach a mammoth $4.6 trillion in 2023 ¹. A subset of this investment will be on tools and technologies needed for Digital transformation which is expected to be $2.3 trillion this year ². However, despite huge investments, the spending only guarantees a little value. In fact, various pieces of research suggest that an average of 87.5% of Digital transformations fail to meet their objective ³. Hewlett-Packard (HP), spent north of $160m on a failed ERP implementation eventually losing $400m in revenues and reportedly leading to a significant shake-up in their executive team ⁴. GE-Digital, a digital transformation business unit by GE had to be spun-off eventually in 2021, after reportedly churning more than $7 billion. A Wall Street Journal analysis stated, “Instead of charging a small team with developing the best product and then letting the operation grow with the product’s evolution, GE set up a huge organization that wasn’t quite needed yet.” ⁵ That is the exact problem and a solution. By setting aside an “IT budget”, and spending millions on technology, Organizations believe in creating a right to succeed for them. Unfortunately, there are more examples of unfinished and expensive IT projects that one can find than the ones delivering any value. CEOs are treating this as an existential crisis⁶ – and rightly so, however, we need to understand that it is not the spending on technology that matters…it is about the business value or potential it can unlock. In my research evaluating organizational spending vs the benefit-driven, I have found certain plans of action or steps that a firm should incorporate in its Digital Strategy. These actions will help avoid this all-or-nothing pitfall and create a sustainable and results-driven digital transformation. Value-first approach As a rule of thumb, measure the business value first and think about IT later. Initiatives, anchored into a business need and value are the only ones that should be part of the Digital Roadmap. American retailer Best-buy, when threatened by Amazon, was forced to re-think its business model and create “a reinvigorated customer experience”. This anchoring to the business need eventually led the company to streamline its warehousing, logistics and software initiatives and become one of the early adopters of a ‘click-and-collect’ model⁶. Core competencies Focus Is digital really your core competency or is it a vehicle to strengthen it? Most firms get entangled between an outcome of a digital initiative and how it relates to its core competency or business model. Emotional attachment to digital initiatives, results in losing sight of the fact that business value is created through a re-invigorated and compelling consumer experience and not via a 3-year-long digital project. Business leaders need to step back and map out, with certainty, what critical expertise their businesses possess to drive digital transformation and which ones they can ‘borrow’ through an external expert. In today’s gig economy, it is becoming increasingly cost-effective and convenient to hire outside talent on short-term and targeted projects. Word of caution, however, is to choose your partners very carefully and based on the alignment of how their core competencies would complement yours. Micro-Digital factories A major problem, the over-committing of resources causes, is the diminishing return on investment (ROI). Firms get trapped in a vicious circle of investing more to create return only to find out each incremental investment further diluting the overall ROI. In 2013, BBC wrote off £98 million in unusable technology assets due to an overly complex digital investment not being governed effectively ⁷. To overcome this dilemma it is critical to have a 3 to 5-year operational boundary around a digital transformation with a clear roadmap of ‘Digital Initiatives’ being delivered within 6-12 months time-frame. However, with a continuous digital flux, the firm’s ability to evolve and pivot with agility throughout this journey, while delivering on specific Digital Initiatives, is the key here. It is pertinent to understand the difference between an overarching ‘Digital Vision’ and a ‘Digital Initiative’. While a ’Vision’ is less volatile, longer-term and encompasses the entire organisation, a ‘Digital initiative’ needs to be delivered in a short, repetitive and agile manner. Small teams of cross-functional experts, developers, and analysts should be structured in the form of ‘Micro-Digital Factories’ at the business unit level, responsible for experimentation and agile delivery of digital initiatives in the form of an MVP (Minimum Viable Product). The self-sharpening and standardized processes need to be defined within these micro-digital factories to ensure faster decision-making on digital ideas and the scalability of initiatives. The results-driven and incremental approach to digital transformation not only creates excitement across the organization as tangible results can be seen in a short time, but it also minimizes the downtime or disruption caused by conventional ‘IT projects’. The correct orchestration of deploying resources while creating maximum ROI requires an agile, value-driven and cost-effective approach. The steps mentioned above can act as a guiding principle to create the right momentum for a firm’s Digital Transformation journey.
Riding the AI Wave > 5 Priorities for CFOs in 2024
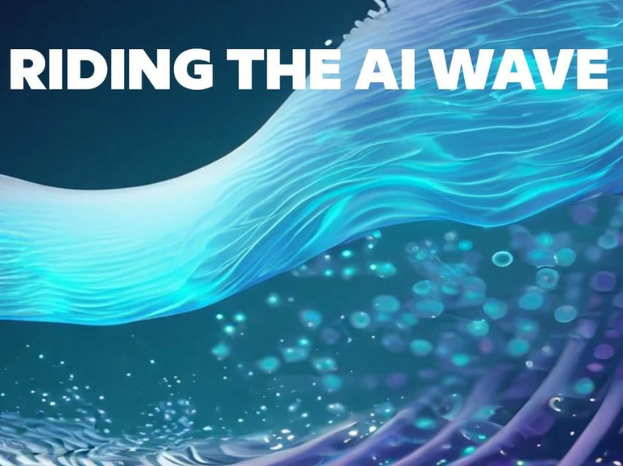
Tariq Munir shares his latest insights with CFO Magazine A/Z on how to create, grow, and lead the Finance function with an AI-First Digital Transformation Strategy in 2024! It will not be an overstatement if we regard 2023 as a year of ‘AI Tectonic Shift’. The seismic activity shook the entire industries and is reshaping the traditional business landscape. It has questioned some of our most basic assumptions regarding the domains that have previously been exclusively human-dominated — creativity and contextual thinking. Experts and thinkers believe that this is just a start. The exponential increase in computing power and the corresponding decrease in cost have made the development, deployment, and widespread adoption of AI models more accessible than ever. In 2006, it would cost around $0.15 to store 1GB of data on Amazon S3…today it costs $0.023 per GB — an 85% decrease in cost. The Finance function, due to its access to organisational data and systems and its ability to influence key decision-making, sits at the centre of the AI disruption. CFOs are playing a vital role in, or in some cases even own, the Digital Transformation agenda of the organisation. On top of this, the VUCA world we live in today has created huge demands and expectations from Finance functions to become strategic business partners and value drivers as opposed to being a conventional accounting and reporting function. 2024 is going to be another year of massive AI growth with a continued convergence of technologies like Generative and Predictive analytics, IoT, cloud computing, and so on. ~Tariq Munir A plethora of AI tools will become available, claiming to solve the issues we face from fast close to scenario planning. On one hand, this requires CFOs to make strategic investments in AI, while on the other they also need to cut through this AI noise and look for the ‘right fit’ technologies as per business needs as opposed to the ‘one-size fits all’ approach. CFOs and their Finance Leadership Teams must set the right priorities for 2024 to address this AI challenge and ride the AI wave successfully. Here are the Five priorities that every Finance leader should have in their “must-win battles” for 2024 Digital Vision and Roadmap Build a clear vision of what a ‘Best-in-class’ Digitally enabled Finance function looks like and create a well-defined and achievable Roadmap. It is critical to think of an ‘AI-first’ strategy aligning with the broader Business Vision and Digital Transformation Strategy. Divide the roadmap into time-bound projects and focus on 2024 to re-base your AI and Digital efforts. There is, however, no need to go all-in. Tackle small incremental projects in agile iterations and prioritize the ones with the highest return on your roadmap. This will help build the case for digitalization and create excitement amongst Finance teams as they will be able to see tangible benefits. Process Optimization CFOs need to focus a sizeable part of their functions efforts to optimize the existing processes and remove the underlying complexities. It is like ‘priming’ the surface before painting. For the AI-first efforts to stick, CFOs must prioritize this. Use a combination of conventional approaches to process optimization augmented with tools like Process and Task Mining. Process optimization creates a virtuous cycle where it helps free up time for Finance teams to find that bandwidth to focus on Digital transformation efforts which in turn creates more hours of productivity. Takeda, a Global Pharmaceutical company, through its “Project Quantum” reduced 75% of manual journals and saved more than 45,000 hours per year globally. Imagine how much time you can get back from removing process complexities and hence in turn enabling you to focus on these priorities. Cybersecurity and Governance With greater digitalization comes more technical complexities and vulnerabilities. Bad actors continue to manipulate loopholes and cost millions to the organization in cyber incidents. As per Statista, the global average cost of one data breach is approximately $4.5million! Rapid and sometimes rushed implementations can create huge susceptibility of financial systems to cyber-attacks. Another factor is the lack of employee awareness and training. In a recent attempt, an organization’s accounting employee was tricked into transferring nearly $87 million to offshore accounts for fake purchases. Therefore, a continuous collaboration with CIOs to ramp up investments in Cybersecurity and having a clear Governance framework around Systems and Data should be a crucial priority for CFOs in 2024. ‘Re-train’ and ‘Retain’ Talent Finding and retaining the right talent is one of the biggest challenges that CFOs will continue to face in 2024. The future of talent is not just core Finance skills. It is a reshaped workforce comprising digitally savvy Finance business partners who can invoke a combination of critical thinking, digital mindset, and curiosity to drive data-driven decision-making. Gartner predicts that by 2026, half of new hires in corporate finance will be non-finance and accounting backgrounds. Upskilling and re-educating the Finance workforce is not a choice anymore. CFOs must invest in providing training and re-training opportunities to build both Digital and Soft skills for their teams in 2024. Again, lead from the front and ensure your leadership team embodies a continuous learning behavior. Apart from classroom style or online training, provide development opportunities by engaging them in exciting AI and Digital projects. Create a best-in-class experience for your finance teams which will not only create engagement but also increase retention as employees increasingly find a purpose and meaning in the work they do. Culture of Psychological Safety Psychologist Carl Rogers coined the term “Psychological Safety” in the 1950s. Rogers defined psychological safety as “the freedom to express oneself without fear of negative evaluation.” If I had to pick only one priority, this is it. A 2017 study by Google found that psychological safety was one of the key factors that distinguished high-performing teams from low-performing teams. Digital Transformation is not possible without experimentation with technologies, incubating new ideas and more importantly — Failing! As finance leaders, building a culture of psychological safety ensures that new ideas are